Generative AI, the seemingly magical technology behind apps like ChatGPT, is made up of two primary functions: AI training and AI inference. Both complement each other and work together to provide users with the best results for chat questions, image requests, and other common generative AI functions.
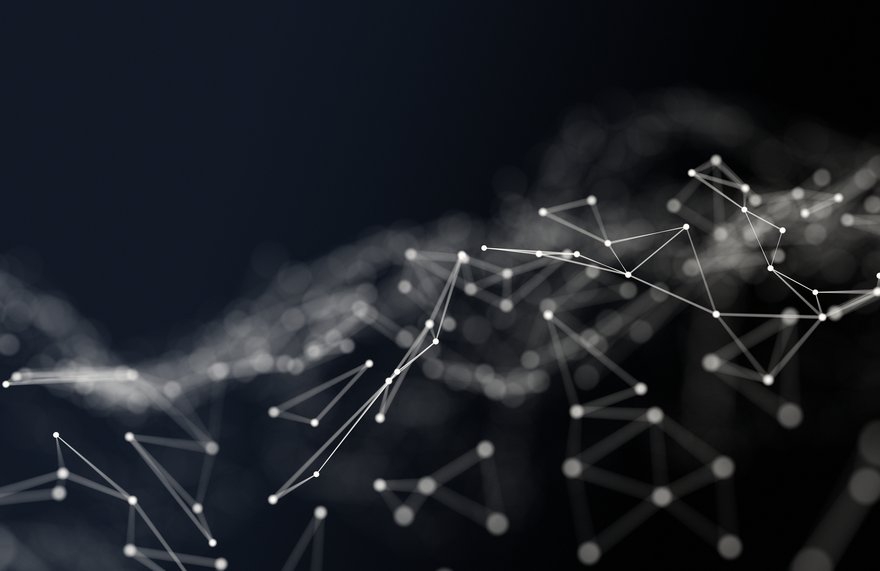
Definition
What is AI training, exactly?
AI training is the first step in the process of building a generative AI application. Training in generative AI refers to the process of giving curated data to algorithms to teach it how to operate.
In a large language model, for example, the model is trained on billions of parameters of text that can include newspapers, websites, online chats, and social media.
Why it's important
Why is AI training important?
To understand why AI training is so important, it might help to look at the evolution of OpenAI's GPT (generative pre-trained transformer), the technology that forms the basis of ChatGPT.
When OpenAI launched, it had 117 million parameters, and its capabilities were much more limited than it is now. GPT-1 was trained on the Common Crawl, a data set of websites across the internet, and the BookCorpus dataset, a collection of 11,000 books. At the time, the technology was groundbreaking, and it was able to produce fluent language based on a prompt (but only in relatively short passages).
By comparison, the latest version of GPT-4 is believed to be trained on trillions of parameters and includes a broader range of data than earlier versions.
Consequently, GPT-4 is much more capable than previous versions, and light years ahead of GPT-1. GPT-4 can interpret images. It can respond to complex prompts and can match human-like abilities in professional tests such as bar exams and grad school entrance tests like the MCAT.
Inference does play a role in the advancement of generative AI tools like ChatGPT, but any model will only be as good as the data it's trained on. The more data that's used in training, the more capable it will be.
How to evaluate
How to evaluate AI training
If you're trying to choose between different AI models, such as chatbots or image generators, you'll need a way to evaluate them, and you'll want to evaluate them on how well they're trained.
There are several different ways you can evaluate AI training models. First, you can see how many parameters they were trained on and the data sources, which should give clues to the model's behavior.
You can also test the different models for accuracy. For example, you can ask them various questions pertaining to history, science, and other subjects to see if the model can answer them correctly. If you are using an image generator, you can use image prompts of increasing detail to test how well a model has been trained and can accurately interpret your prompt.
Other factors you can use to evaluate AI training include precision -- or how specific an answer is -- and recall, which means the model's ability to remember the questions that have already been asked and to respond contextually.
Related investing topics
Example
An example of how AI training works
One relatively simple example of how AI training works is with online recommendations.
For example, sites like Amazon (AMZN 0.58%), Netflix (NFLX 1.73%), and YouTube will recommend products, shows, or videos based on previous patterns from other users or based on your personal preferences.
This is a form of machine learning, but it's also another way that AI is trained since the model will adapt based on your choices and those of future users. Those choices become relevant data for the model.
AI models are typically trained with a small dataset to start, expanding the data over time as the model grows more sophisticated.
In other words, training AI models isn't all that different from teaching humans, using increasing amounts of information so they can make inferences as they progress.
As training techniques become more sophisticated and datasets become even larger, generative AI models should continue to get better, and training will remain a key component to building these transformative models.